Exploring the future frontiers of NLP research (paper review)
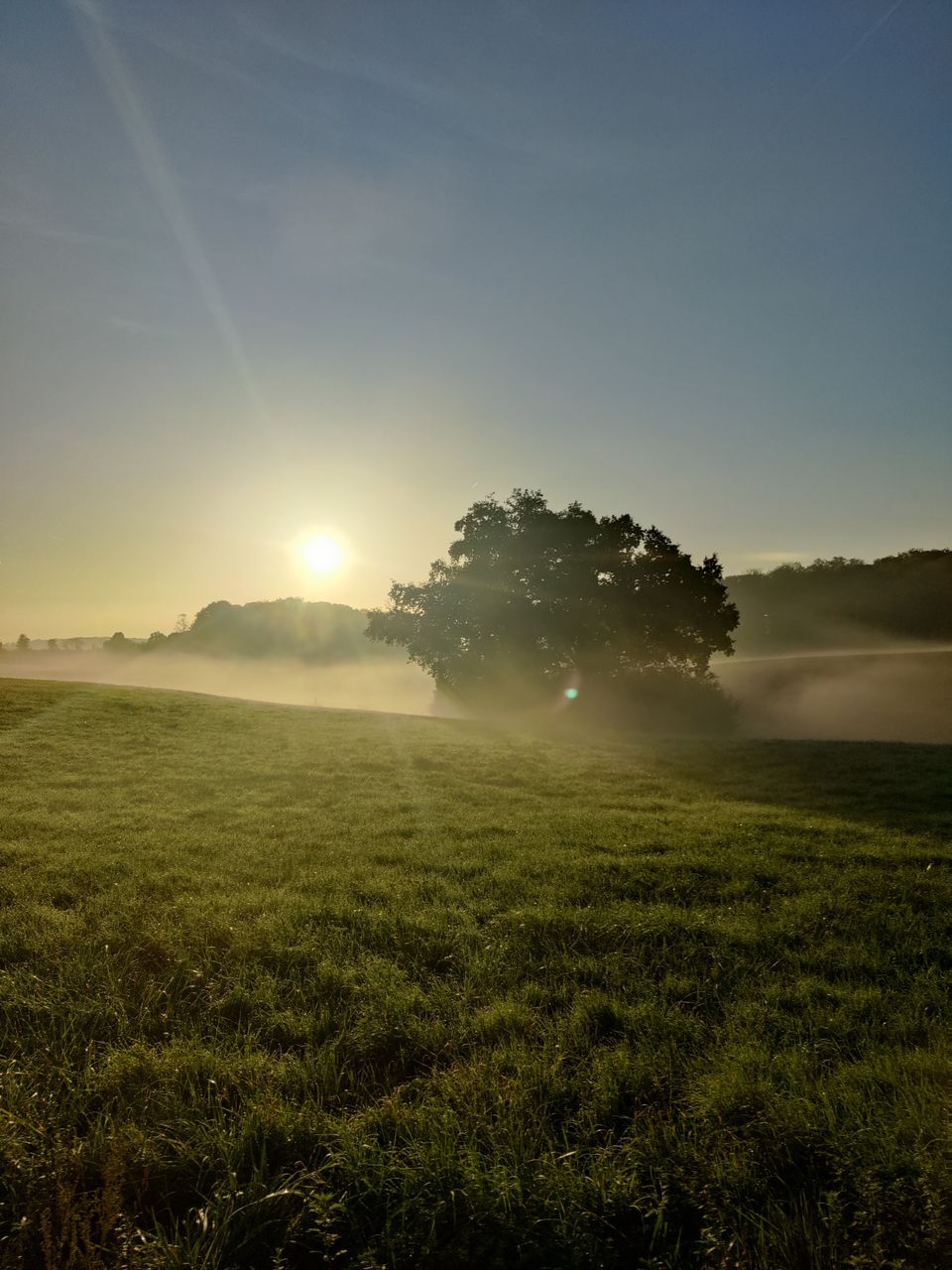
Paper: https://arxiv.org/abs/2305.12544
Title: A PhD Student's Perspective on Research in NLP in the Era of Very Large Language Models
Authors: Oana Ignat, Zhijing Jin, Artem Abzaliev, Laura Biester, Santiago Castro, Naihao Deng, Xinyi Gao, Aylin Gunal, Jacky He, Ashkan Kazemi, Muhammad Khalifa, Namho Koh, Andrew Lee, Siyang Liu, Do June Min, Shinka Mori, Joan Nwatu, Veronica Perez-Rosas, Siqi Shen, Zekun Wang, Winston Wu, Rada Mihalcea
This blog post offers a summary of a paper that discusses the future of Natural Language Processing (NLP) research, which I came across last week (it was published 21 May 2023). Highlighting the significance of language and people, the authors propose various compelling directions for NLP research projects. From multilinguality and low-resource languages to reasoning, knowledge bases, language grounding, computational social sciences, NLP for online environments, child language acquisition, non-verbal communication, synthetic datasets, interpretability, efficient NLP, NLP in education and healthcare, and NLP ethics – each topic reveals exciting opportunities and challenges.
It is definitely a timely discussion because the rapid development of large language models (LLMs) in the recent years has made many people wonder which directions NLP research should now take. As a linguist, I particularly appreciated that the authors remind us about the importance of the word "language" in "natural language processing". Large language models is not everything: we still have so many interesting topics to explore!
Multilinguality and low-resource languages
The NLP research and community lack representation of numerous languages and cultural/linguistic backgrounds. While we generate extensive multilingual data, language models mostly focus on dominant languages such as English. To mitigate the underrepresentation of diverse linguistic and cultural contexts in NLP, it is crucial to develop language models for low-resource languages and integrate code-switching data into the research.
Reasoning
Can artificial intelligence emulate human reasoning? Exploring avenues such as integrating external knowledge sources, moral reasoning, and evaluating the reasoning skills of models are potential research directions in this pursuit.
Knowledge bases
Language models exhibit a phenomenon known as hallucination, wherein they generate nonsensical but seemingly plausible outputs due to the lack of external grounded knowledge, absence of database connections, and a deficiency in general cultural and common sense. Additionally, as models are predominantly trained on data representing dominant Western cultures and languages, incorporating external knowledge bases to encompass global cultural and linguistic diversity presents a significant challenge for future NLP researchers.
Language grounding
This research direction focuses on establishing connections between natural language and the physical world. It involves multimodal models that engage in tasks like visual question answering, image and video captioning, and text-to-image retrieval and generation. The authors also explore lesser-known combinations of modalities, such as those used in detecting alertness levels, identifying depression, or uncovering deceptive acts. This requires bridging the gap between verbal expressions and physiological, sensory, and behavioral modalities, which have received less attention compared to image and video formats. Additionally, I am acquainted with someone involved in a research project aimed at developing a personal assistant capable of recognizing Australian Sign Language and generating signed responses through an avatar. This project is fascinating and aligns with the human-centric approach that AI projects should embrace.
Computational social sciences
I worked in this field, also known as digital humanities, as a PhD researcher in 2017-2021. Back then, topic modeling and word embeddings were very new and exciting method to use. What will be the future trends? There is a need for innovative approaches in digital humanities, making it an intriguing and practical area of research. Moreover, the issue of disproportionately focusing on dominant languages and cultures in data analysis is also prevalent in computational social sciences research.
NLP for online environments
The vast volume of digital content generated by humans every second, particularly in online communities and social networks, presents two primary research directions: content moderation and content generation. NLP can play a role in monitoring and analysing user-generated content to detect and prevent misinformation, manipulation, and other malicious uses of language in online environments. It is essential to ensure that content moderation is conducted in a fair manner that allows all voices to be heard. Regarding content generation research, a promising direction involves identifying the individuals behind the content and discerning the interests it aims to promote. Ultimately, these efforts revolve around ensuring internet safety and promoting fairness.
Child language acquisition
If we want to create language models showing signs of artificial general intelligence (AGI), we should take inspiration from children who effortlessly pick up multiple languages. Take my son, growing up in multilingual Luxembourg with three national languages and two additional languages at home. He learned them all without breaking a sweat, even though he had access to technically limited datasets (aka everyday conversations). So, how can we achieve this data efficiency in language model learning? Studying how kids learn languages might provide insights, but researching children comes with its fair share of challenges—both practical and ethical.
Non-verbal communication
There is another interesting avenue to explore: the integration of non-verbal communication such as gestures, facial expressions, sign language, and even emojis. By infusing these non-verbal elements into existing language representations, we can enhance their richness and depth. This research direction encompasses various aspects, including the interpretation of non-verbal language, understanding sign language, generating or translating non-verbal cues, and fostering effective communication that blends verbal and non-verbal signals. This captivating theme of exploration aligns, to some extent, with the concept of language grounding we previously discussed. It offers an opportunity to delve deeper into the intricacies of human communication and the potential for NLP to capture its nuances.
Synthetic datasets
In situations where data collection is impractical, prohibitively expensive, or hindered by privacy concerns, an alternative approach is to leverage synthetic data created by a generative language model. This allows data scientists to utilise tools like chatGPT to quickly and conveniently create annotated datasets. However, it is crucial to acknowledge that the synthetic data may inherit biases present in the training data used by the model. As a result, ensuring data quality becomes a vital concern, particularly because there are no established evaluation metrics. Moreover, the synthetic data might lack diversity. To address these challenges, further research is needed to explore approaches such as knowledge distillation, exerting control over attributes of the generated data, and transforming existing datasets to create novel ones (for example, with a different style, modalitiy, or format).
Interpretability
Making language models more transparent - and their decisions more justifiable - is a very urgent topic, especially considering the AI legislation, already in force or in the pipeline. For linguists, probing may be the most interesting direction: how can we design a task for a model to reveal the linguistics and world knowledge, as well as biases encoded in it and its reasoning skills? Other ways to improve model interpretability include human-in-the-loop (incorporating human feedback or generating interactive explanations for the model's outputs) and uncovering the under-the-hood mechanisms of the model's decision-making processes.
Efficient NLP
Training large language models requires a lot of computational power and is very energy-consuming, which leads to environmental impacts of the industry. The authors suggest looking at this problem from several angles: data efficiency (how to improve performance of models trained on smaller data), model design (improving attention mechanisms), and efficient downstream task adaptation.
NLP in Education and Healthcare
What seems to unite these two industries in context of NLP applications is the personalisation aspect - a very important aspect of human-centric NLP. Besides, in both education and healthcare, there is no language diversity. Finally, both the education and health applications of NLP lack evaluation methods, making it hard to estimate the systems' real-world impact. What I find particularly fascinating is that in healthcare, natural language processing is seen as capable to contribute to drug discovery through information extraction and analysis - this is the level of interdisciplinary we probably could not even think about some years ago!
NLP and Ethics
There are many ways NLP models and applications can be harmful although they are generally developed to benefit people. This is why research into ways to prevent misuse of NLP is necessary and important as never before. Potential research directions include developing fact-checkers to prevent misinformation or exploring NLP system limitations and loopholes using adversarial NLP. We also need to find ways to debias NLP systems and evaluate how fair they are. Finally, developing methods to ensure maximum data privacy is essential, too, especially if we want to move to personalised NLP systems in such fields as education and healthcare where there is a lof sensitive personal information to protect.
Final thoughts
I am very grateful to the authors of this paper for bringing to light all these fascinating research topics and hope that we will soon see some exciting projects exploring each of the directions discussed. NLP research landscape is evolving and the development of large language models is not killing it but rather changing the direction, offering new opportunities and, as the authors of the paper conclude, now is the best time to return to the linguistics perspectives in NLP and "acknowledge that NLP is about language and people and should be fundamentally human-centric". I think that the focus today should be indeed on how to build NLP systems that benefit humans - which may take form of supporting human decision-making or enhancing human experience in education, at a workplace, or in some other environment - and are created with humans' active involvement ('human-in-the-loop' paradigm). Notably, the lack of cultural and linguistic diversity seem to be a red thread running through many of the research topics discussed in this paper. To ensure that future language models and NLP systems encompass the diverse range of languages and cultures found across humanity, a highly interdisciplinary approach is imperative.
Member discussion